决策树和随机森林
决策树 (Decision Tree) 是一种特殊的树结构,代表的是样本特征与样本标签之间的一种映射关系。
决策树举例如下,分叉路径则代表某个可能的属性值,而终结节点代表最终决策:
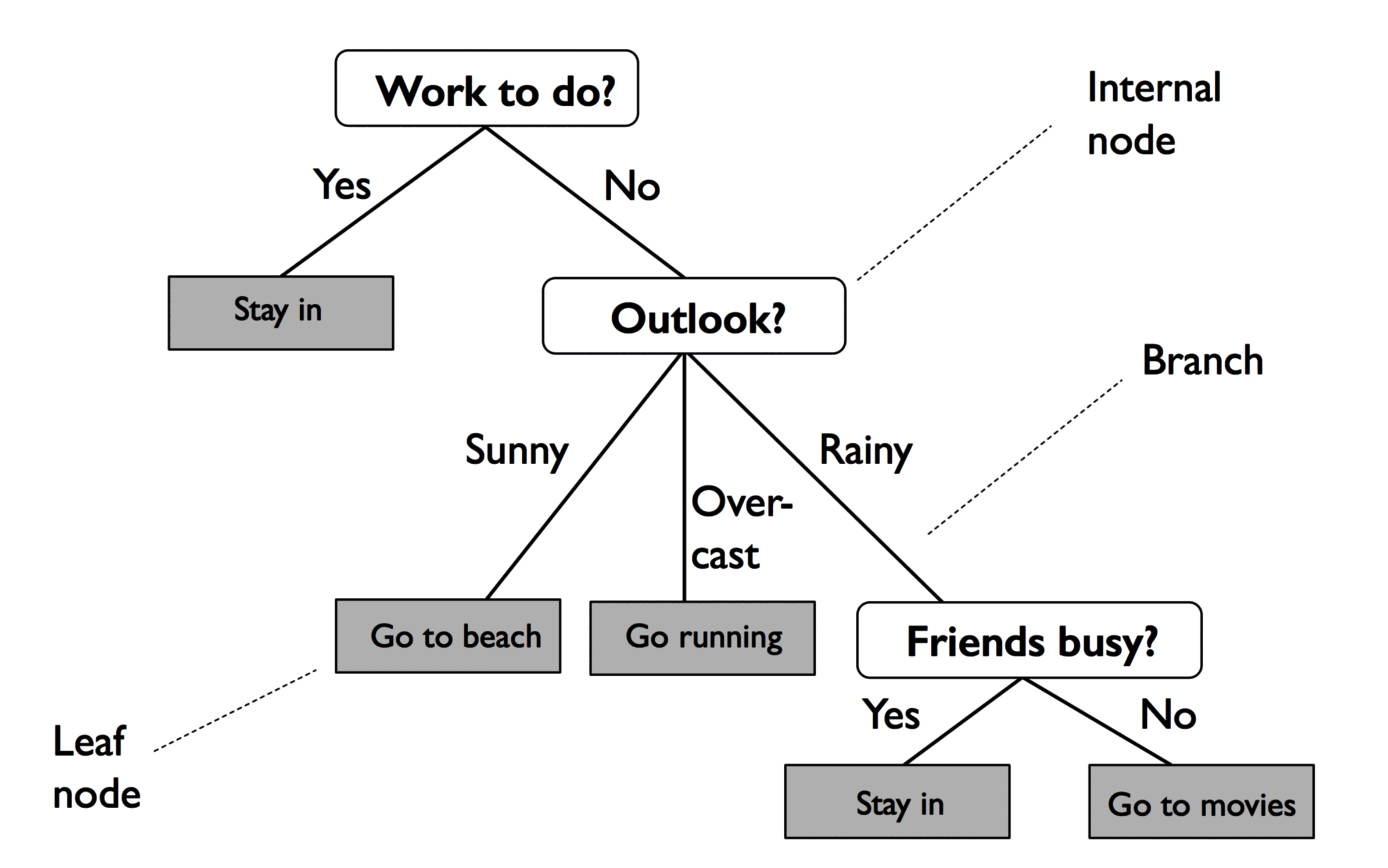
信息增益
定义
为了引入决策树分类的算法,先介绍决策树学习中的重要概念:信息增益 (Information Gain)。
这个指标可以衡量分类给数据中带来信息的程度。若分类带来的信息增益越大,就越能减少数据的无序度。
信息增益的表示如下,为父节点和子节点中的不纯度 (Inpurity) 差值:
其中 代表对应的特征, 和 分别表示父节点的数据和第 个子节点的数据集, 和 分别表示父节点的数据和第 个子节点的样本数量, 代表不纯度的函数。
不纯度的计算有以下几种方法:
- 熵 (Entropy):
- Gini 不纯度 (Gini Impurity):
- 分类错误 (Classification Error):
在实际应用中,熵和 Gini 不纯度的结果非常相似,不必纠结于这两个计算标准的选择。而分类错误对节点概率的变化较不敏感,不推荐在决策树增长时使用。
计算示例
以如下决策树演示不纯度的计算,其中 代表节点中所属分类的样本数量。
例如父节点,包含 40 个属于类 1 的样本、40 个属于类 2 的样本;其他节点同理。
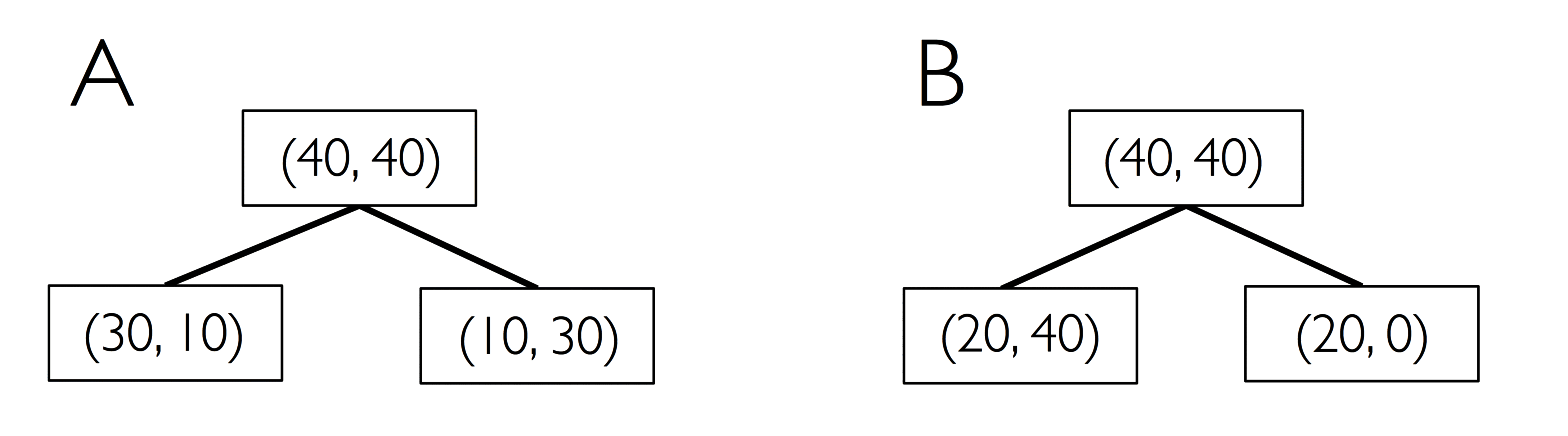
决策树 A 的熵:
决策树 B 的熵:
决策树 A 的 Gini 不纯度:
决策树 B 的 Gini 不纯度:
决策树 A 的分类错误:
决策树 B 的分类错误:
为了更直观的比较三种信息增益计算方法的差异,对平均分布 的样本应用并绘图:
import matplotlib.pyplot as plt
import numpy as np
def gini(p):
return (p) * (1 - (p)) + (1 - p) * (1 - (1 - p))
def entropy(p):
return -p * np.log2(p) - (1 - p) * np.log2((1 - p))
def error(p):
return 1 - np.max([p, 1 - p])
x = np.arange(0.0, 1.0, 0.01)
ent = [entropy(p) if p != 0 else None for p in x]
sc_ent = [e * 0.5 if e else None for e in ent]
err = [error(i) for i in x]
ax = plt.subplot(111)
for (
i,
lab,
ls,
c,
) in zip(
[ent, sc_ent, gini(x), err],
["Entropy", "Entropy (scaled)", "Gini Impurity", "Misclassification Error"],
["-", "-", "--", "-."],
["black", "lightgray", "red", "green", "cyan"],
):
line = ax.plot(x, i, label=lab, linestyle=ls, lw=2, color=c)
ax.legend(
loc="upper center", bbox_to_anchor=(0.5, 1.15), ncol=5, fancybox=True, shadow=False
)
ax.axhline(y=0.5, linewidth=1, color="k", linestyle="--")
ax.axhline(y=1.0, linewidth=1, color="k", linestyle="--")
plt.ylim([0, 1.1])
plt.xlabel("p(i=1)")
plt.ylabel("Impurity Index")
plt.show()
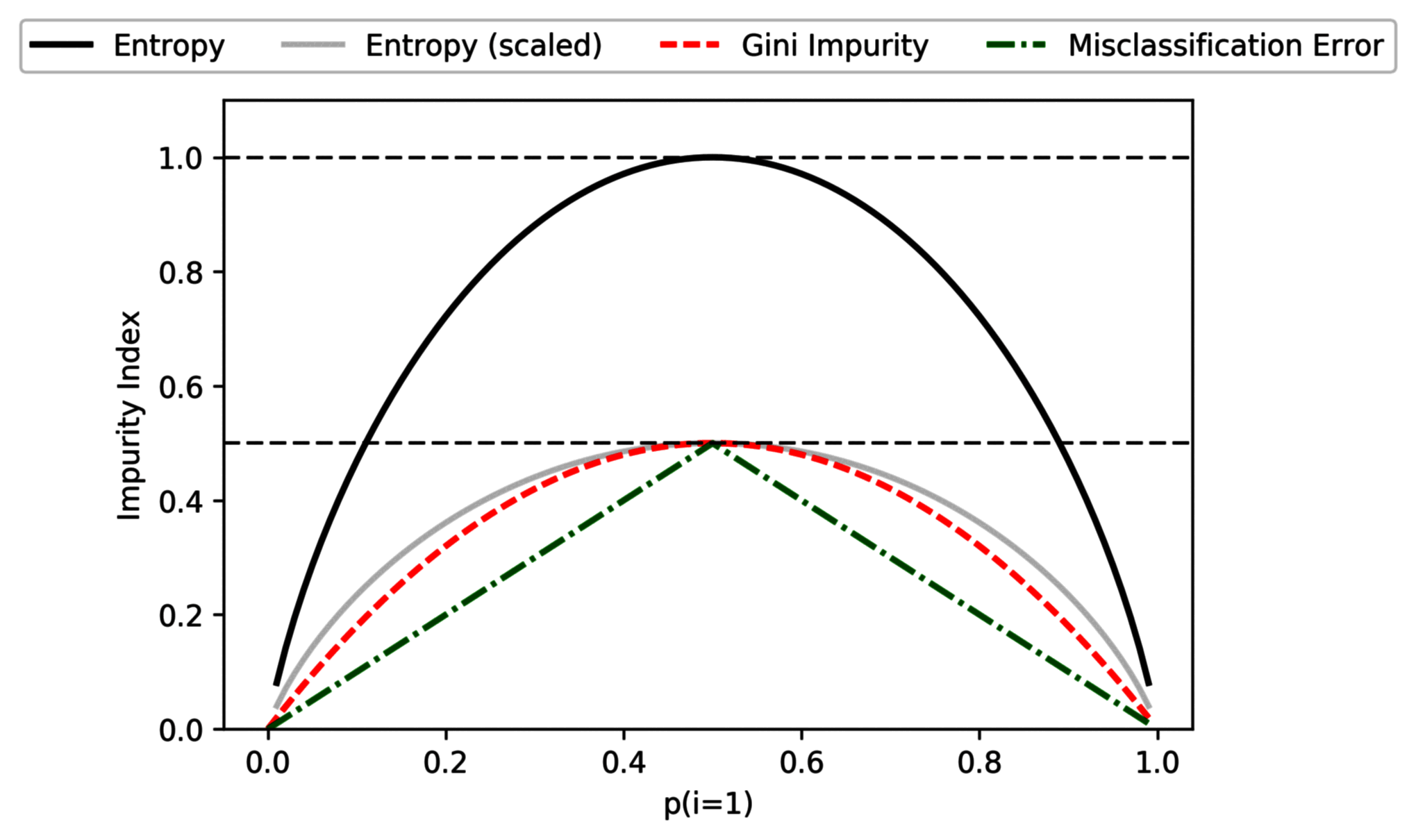
决策树
代码实现
决策树分类的思想是,从树根开始将特征上的数据分割成最大的信息增益,然后在子节点重复这个拆分过程。
在实践中,这可能会导致一个有很多节点的很深的树,很容易导致过度拟合。因此通常要通过设置树的最大深度限制来修剪树。
以 Iris 数据集为例,基于 Scikit-learn 的实现如下 (其中决策边界绘制函数 plot_decision_regions
请参见之前的文章):
import matplotlib.pyplot as plt
import numpy as np
from sklearn import datasets
from sklearn.model_selection import train_test_split
from sklearn.tree import DecisionTreeClassifier
iris = datasets.load_iris()
X = iris.data[:, [2, 3]]
y = iris.target
X_train, X_test, y_train, y_test = train_test_split(
X, y, test_size=0.3, random_state=1, stratify=y
)
tree = DecisionTreeClassifier(criterion="gini", max_depth=4, random_state=1)
tree.fit(X_train, y_train)
X_combined = np.vstack((X_train, X_test))
y_combined = np.hstack((y_train, y_test))
plot_decision_regions(X_combined, y_combined, classifier=tree, test_idx=range(105, 150))
plt.xlabel("petal length [standardized]")
plt.ylabel("petal width [standardized]")
plt.legend(loc="upper left")
plt.show()
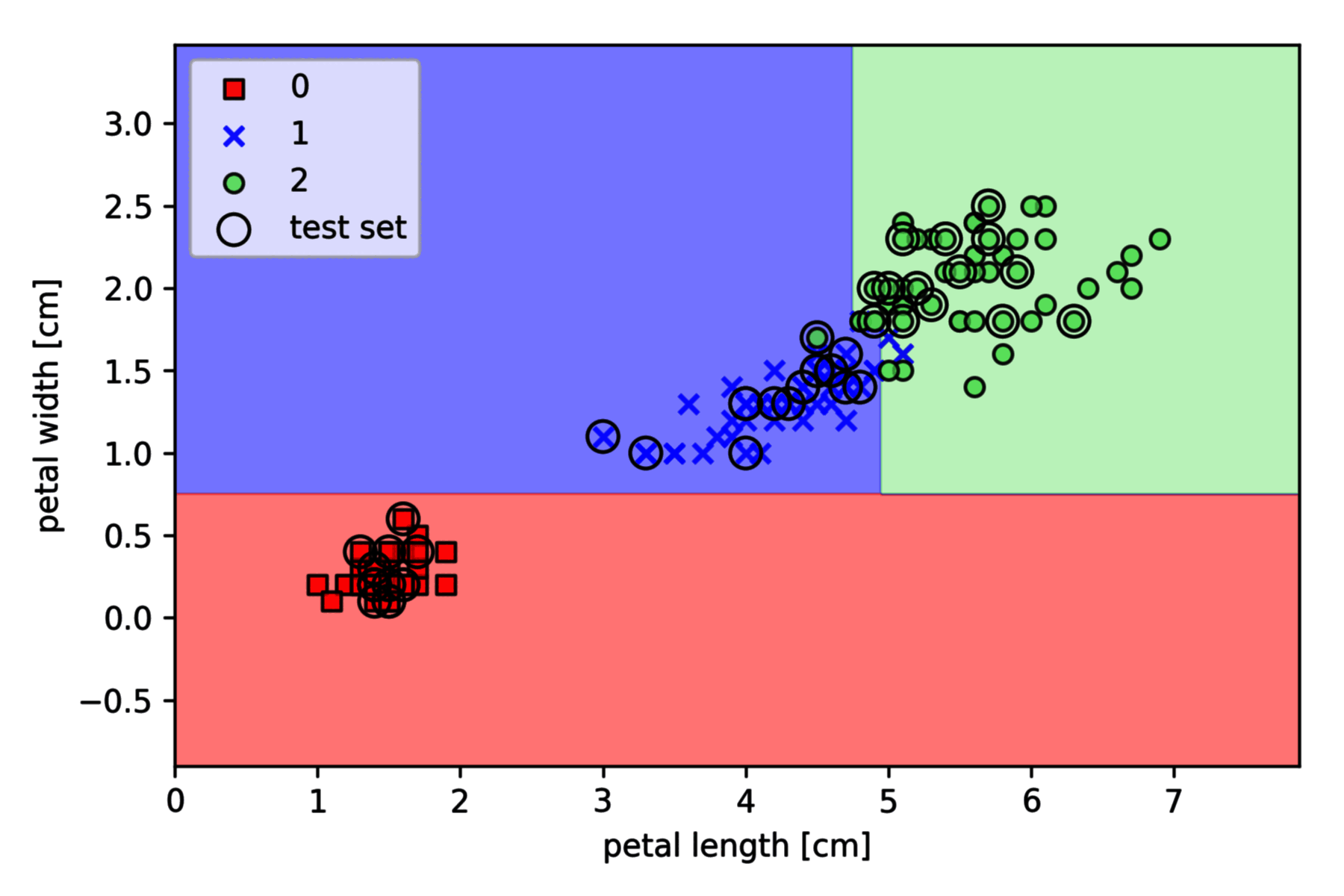
Graphviz 绘图
Windows 下的 Graphviz 需要:
- 下载并安装 Graphviz Windows 安装包
graphviz-2.xx.msi
- 将
C:\Program Files (x86)\Graphviz2.xx\bin\;
添加到环境变量Path
(计算机
——属性
——高级系统设置
——环境变量
) - Pip 安装软件包:
pip install pydotplus graphviz pyparsing
对上节生成的 tree
进行绘图:
from pydotplus import graph_from_dot_data
from sklearn.tree import export_graphviz
dot_data = export_graphviz(
tree,
filled=True,
rounded=True,
class_names=["Setosa", "Versicolor", "Virginica"],
feature_names=["petal length", "petal width"],
out_file=None,
)
graph = graph_from_dot_data(dot_data)
graph.write_png("tree.png")
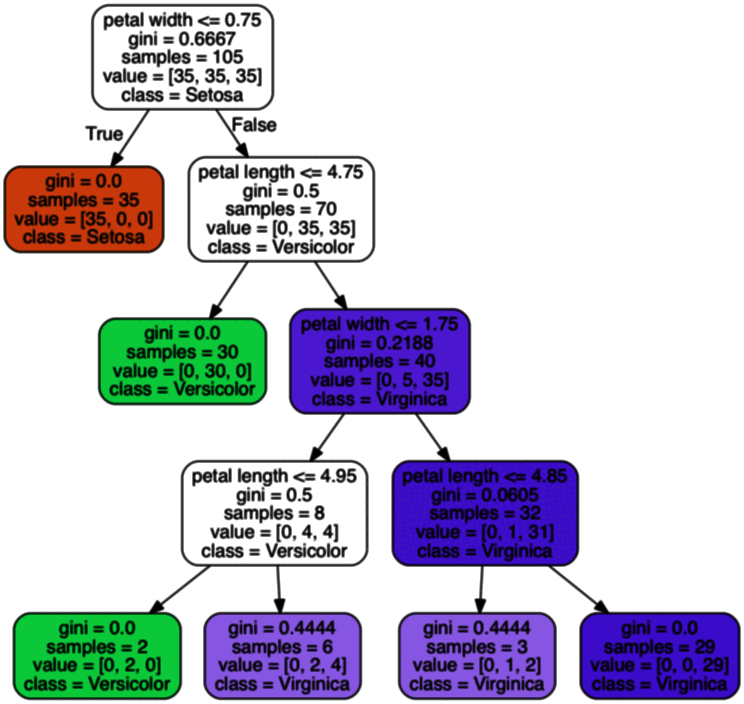
随机森林
随机森林是一个包含多个决策树的分类器,并且其输出的分类标签是由每个树的的分类标签的众数而定。
- 从已知的样本集合 (样本数 ,特征数 ) 中,有放回地随机取 个 bootstrap 样本 ()。
- 对取得的 bootstrap 的样本生成决策树:a。无放回地随机选择其中 个特征 ()。b。根据目标函数提供的最佳分割特征 (如最大化信息增益) 来分割节点。
- 重复步骤
1-2
若干次数。 - 汇总每棵树的预测标签,把其中数量最多的作为随机森林的分类标签。
import matplotlib.pyplot as plt
import numpy as np
from sklearn import datasets
from sklearn.model_selection import train_test_split
from sklearn.ensemble import RandomForestClassifier
iris = datasets.load_iris()
X = iris.data[:, [2, 3]]
y = iris.target
X_train, X_test, y_train, y_test = train_test_split(
X, y, test_size=0.3, random_state=1, stratify=y
)
forest = RandomForestClassifier(
criterion="gini", n_estimators=25, random_state=1, n_jobs=2
)
forest.fit(X_train, y_train)
X_combined = np.vstack((X_train, X_test))
y_combined = np.hstack((y_train, y_test))
plot_decision_regions(
X_combined, y_combined, classifier=forest, test_idx=range(105, 150)
)
plt.xlabel("petal length [standardized]")
plt.ylabel("petal width [standardized]")
plt.legend(loc="upper left")
plt.show()
上面通过 n_estimators
参数从 25 个决策树中训练了一个随机森林,并使用 Gini 不纯度作为分割节点的标准。n_jobs
参数表示可以使用计算机的多个核心 (这里是两个核心) 来并行化训练。
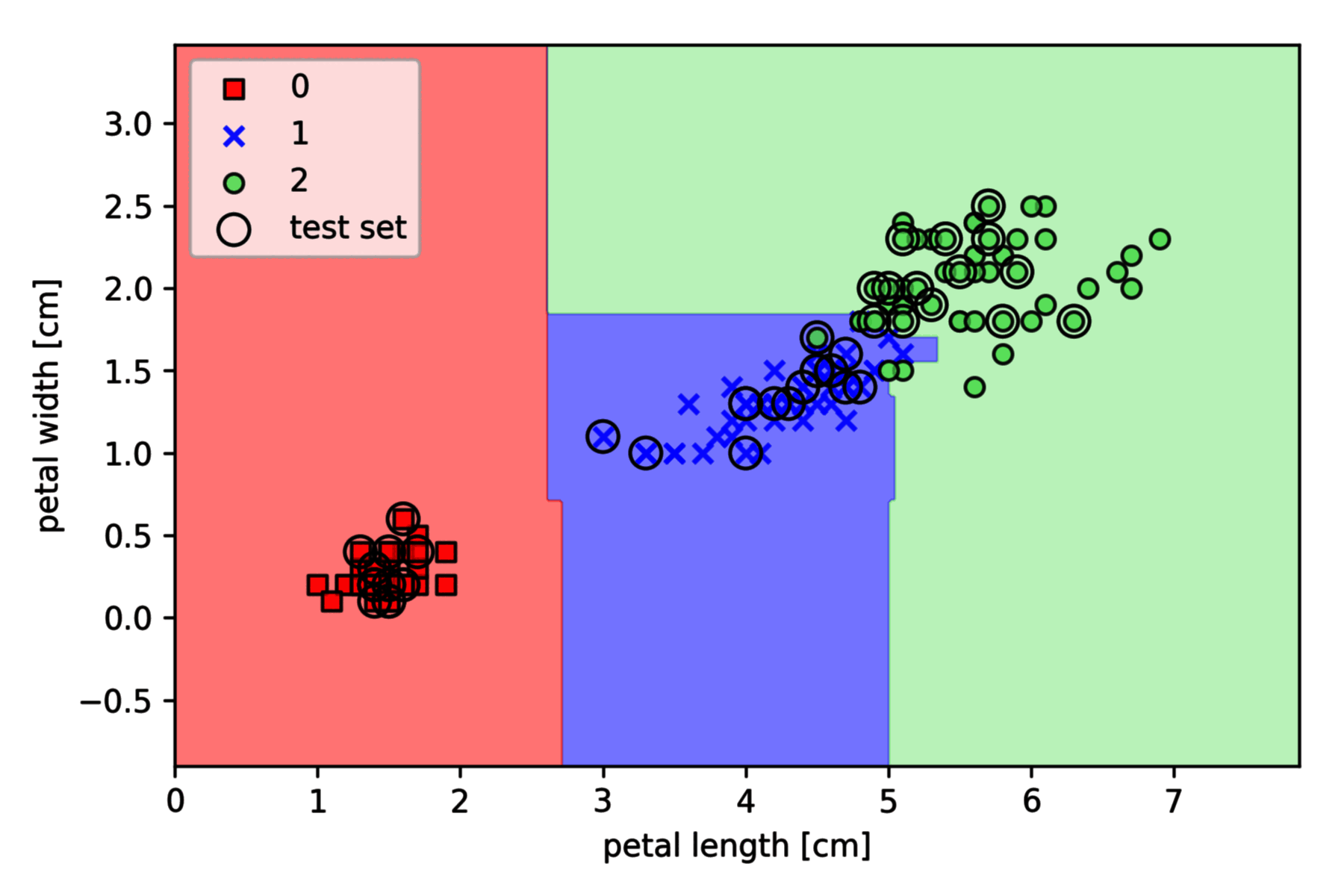